MCP: Building Your SecOps AI Ecosystem
How the open Model Context Protocol creates new possibilities for connecting AI with enterprise security tools.
Introduction
Security operations teams have spent years trying to build the perfect integration layer between their tools and workflows. We've gone from manual API scripts to elaborate SOAR platforms, yet most security analysts still jump between countless tabs and interfaces during investigations. While generative AI has reshaped how we interact with data, connecting these powerful models to our security tooling has remained a developer's challenge—until now.
Enter Model Context Protocol (MCP), an open paradigm for orchestrating connections between large language models and the everyday tools used by developers and security teams. Unlike traditional API integrations that require custom code for each connection, MCP provides a standardized way for LLMs to interact with tools like Google Drive, Box, and dozens more. While MCP was designed as a general-purpose protocol, it represents a crucial opportunity to accelerate security operations automation - providing a standardized way for security teams to integrate AI capabilities across their entire toolchain without writing custom code for each tool.
In this post, we'll explore MCP's potential in security operations, how it compares to purpose-built agents, and what security teams need to know to start leveraging this powerful new approach.
Understanding MCP: The New Paradigm
MCP was developed and open-sourced by Anthropic in late 2024:
Model Context Protocol (MCP) is a new standard for connecting AI assistants to the systems where data lives, including content repositories, business tools, and development environments. Its aim is to help frontier models produce better, more relevant responses.
At its core, MCP (Model Context Protocol) is a specification that standardizes how language models interact with external tools and data sources. Think of it as the "HTTP of AI"—a common language that allows LLMs to communicate effectively with backend services and APIs. MCP’s popularity surged this past week when Sam Altman announced that OpenAI would begin adding support for MCP across their products:
This marks a significant step toward LLM ecosystem interoperability, which has major implications for security operations. After all, effective security depends on connecting enterprise-wide data, security tools, and teams to gain a comprehensive view.
MCP Architecture
MCP uses a server-client architecture in which servers provide capabilities that LLMs can access through client applications. While servers typically run as local web services, cloud-hosted vendor solutions are becoming more common.
This flexible architecture has led to a growing ecosystem of specialized clients:
Cursor for security code development and analysis
Claude Desktop for general security conversations and analysis
Goose for automating complex development tasks
This separation between clients and the underlying protocol creates a dynamic environment where innovation can occur independently at both levels—new clients can emerge without disrupting existing tools, and new tools can be added without requiring client updates.
An MCP server consists of three key components:
Tools: The functional building blocks are discrete capabilities that perform specific actions when called.
Prompts: Reusable templates that guide LLM interactions with tools and users, ensuring consistent and effective responses across common scenarios.
Resources: Static or dynamic data sources that tools and prompts can reference, enriching the capabilities of both LLMs and tools.
These components work in concert to help the LLM complete user tasks while incorporating relevant external context.
Example MCP Server
Let’s examine a quick example of a SIEM-based MCP server with tools such as log source onboarding, writing detection rules, querying underlying log data, and listing and triaging alerts.
Here’s an example list_alerts
tool interface with various arguments for the LLM to utilize.
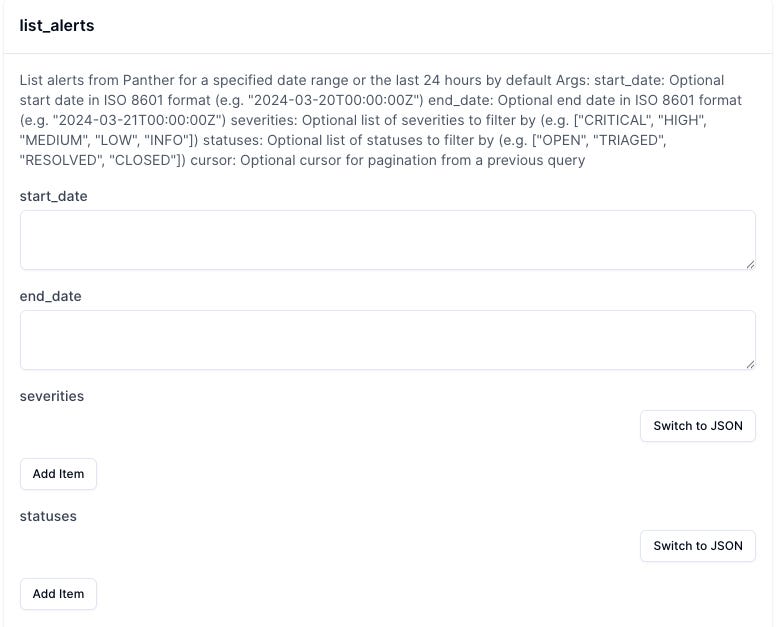
When this MCP server is installed on the system, configured Clients start new sessions/chats, connect to the server, and begin utilizing tools automatically based on user requests, like asking to summarize all alerts created on a particular date:
Permissions tend to be handled by the Clients themselves. For example, Claude Desktop explicitly asks users for permission before granting access to specific tools and servers, which is reassuring for privacy purposes:
Ad-Hoc vs. Predefined Workflows
MCP's flexibility allows it to support both ad-hoc exploration and predefined workflows. When dealing with novel investigations or unique scenarios, security teams can leverage a conversational interface to step through investigations with natural dialogue while the LLM selects and combines tools based on the context. For instance, an investigation might begin with questions about suspicious network traffic, naturally progress to examining the involved hosts, and then pivot to analyzing user activities—all flowing naturally without rigid, predefined patterns.
On the other hand, MCP also excels at handling routine, standardized processes through predefined, structured prompts that guide the LLM through specific sequences of tool usage. A perfect example is the "New Phishing Email Assessment" workflow, where the LLM systematically extracts and analyzes URLs and attachments, verifies sender reputation and authenticity, searches for similar patterns in recent emails, and provides recommended response actions.
What sets this apart from traditional automation is the maintained flexibility—even within these predefined workflows, the LLM can adapt its approach based on discoveries made during the assessment process.
MCP vs. Purpose-Built Agents
We have previously written about the advent of AI agents for SecOps and how they can significantly improve team efficiency and execution quality, keep humans in the loop for critical decisions, and enable them to focus on higher-order strategic initiatives. AI agents and MCPs serve distinct yet complementary roles in the security ecosystem. Understanding their fundamental differences helps security teams deploy each solution where it provides the most value.
Purpose-Built Agents: Problem Solvers
Purpose-built security agents are designed to solve specific problems end-to-end. They're highly specialized systems optimized for particular security functions:
Alert triage agents that automatically investigate and classify security alerts
Detection engineer agents that threat model and write code to cover various cases
Threat-hunting agents that proactively search for indicators of compromise
These agents excel when:
The problem domain is well-defined
The workflow follows predictable patterns
Speed and consistency are critical
Minimal human intervention is preferred
Purpose-built agents are essentially "solutions" rather than "tools." They encapsulate security expertise into automated workflows with precise inputs and outputs.
MCP: Tool Orchestrator
In contrast, MCP is a flexible orchestration layer that empowers LLMs to access and control various tools based on context and user guidance. MCP doesn't solve specific problems; it enables dynamic tool selection and usage.
MCP shines when:
Workflows can't be fully predicted in advance
Multiple tools need to be coordinated
Human guidance drives the investigation
Flexibility and exploration are priorities
If purpose-built agents are like specialized robots designed for specific tasks, MCP is more like a universal remote control that can operate any device in your security arsenal.
Benefits of MCP for Security Teams
The adoption of MCP in security operations delivers several compelling advantages:
Time Savings and Efficiency
The most immediate benefit is the elimination of context switching. Security analysts typically use 20+ tools during investigations, and the cognitive load of moving between systems significantly impacts productivity. By centralizing access through natural language interfaces, MCP can reduce investigation time by 30-50%, allowing analysts to focus on analysis rather than data gathering.
Integration into the Security Ecosystem
Unlike proprietary automation platforms that create walled gardens, MCP is designed for interoperability. Its standardized approach means that security teams can connect virtually any tool with an API—from commercial security products to internal custom applications. This universality eliminates the "integration tax" that has traditionally limited the effectiveness of security automation.
Control Over Models and Prompts
MCP gives security teams unprecedented control over which LLMs they use for different tasks. Teams can select models based on their specific requirements:
Open-source models for sensitive internal data
Specialized security models for technical analysis
General-purpose models for natural language tasks
This flexibility allows security organizations to optimize for cost, performance, or data privacy based on their unique needs rather than being locked into a vendor's model choices.
The Emerging Introduction of MCP into SecOps
As MCP continues to mature, we anticipate several developments in the security landscape:
Pre-built security integrations: Major security vendors will release native MCP connectors, making implementation seamless.
Security-focused standards: The protocol will evolve to address security-specific data sensitivity and compliance needs.
Unified agent architecture: Purpose-built agents and MCP will converge, combining specialized automation with flexible orchestration.
By standardizing how AI interfaces with security tools, MCP breaks down the integration barriers that have historically limited automation and efficiency in security operations.
While purpose-built agents will continue excelling at specific tasks, MCP provides the flexible orchestration layer for complex, human-guided investigations. Forward-thinking security teams will leverage both approaches, maximizing their strengths while building a more integrated security ecosystem. Early adopters of MCP should set realistic expectations and start small, focusing on well-defined use cases while building expertise. However, the investment in MCP capabilities today will position teams for success as AI continues to reshape security operations.
The most effective security programs of tomorrow will be those that successfully blend human expertise, specialized agents, and MCP-enabled tool orchestration. This powerful combination promises to deliver security capabilities that far exceed what any single approach could achieve alone.